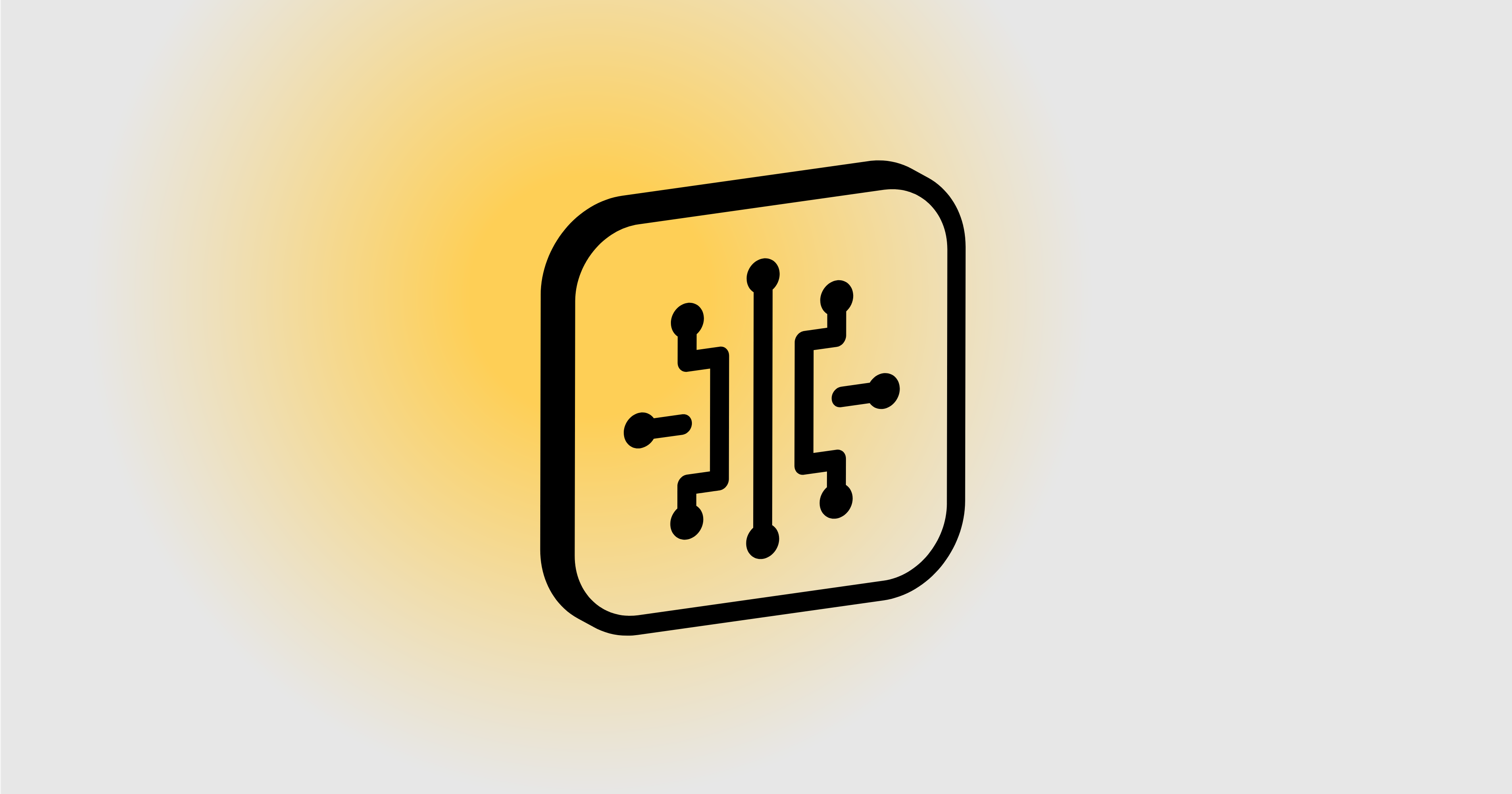
AI & Machine Learning Consulting for Businesses
Transform your data with AI and ML solutions tailored to your business
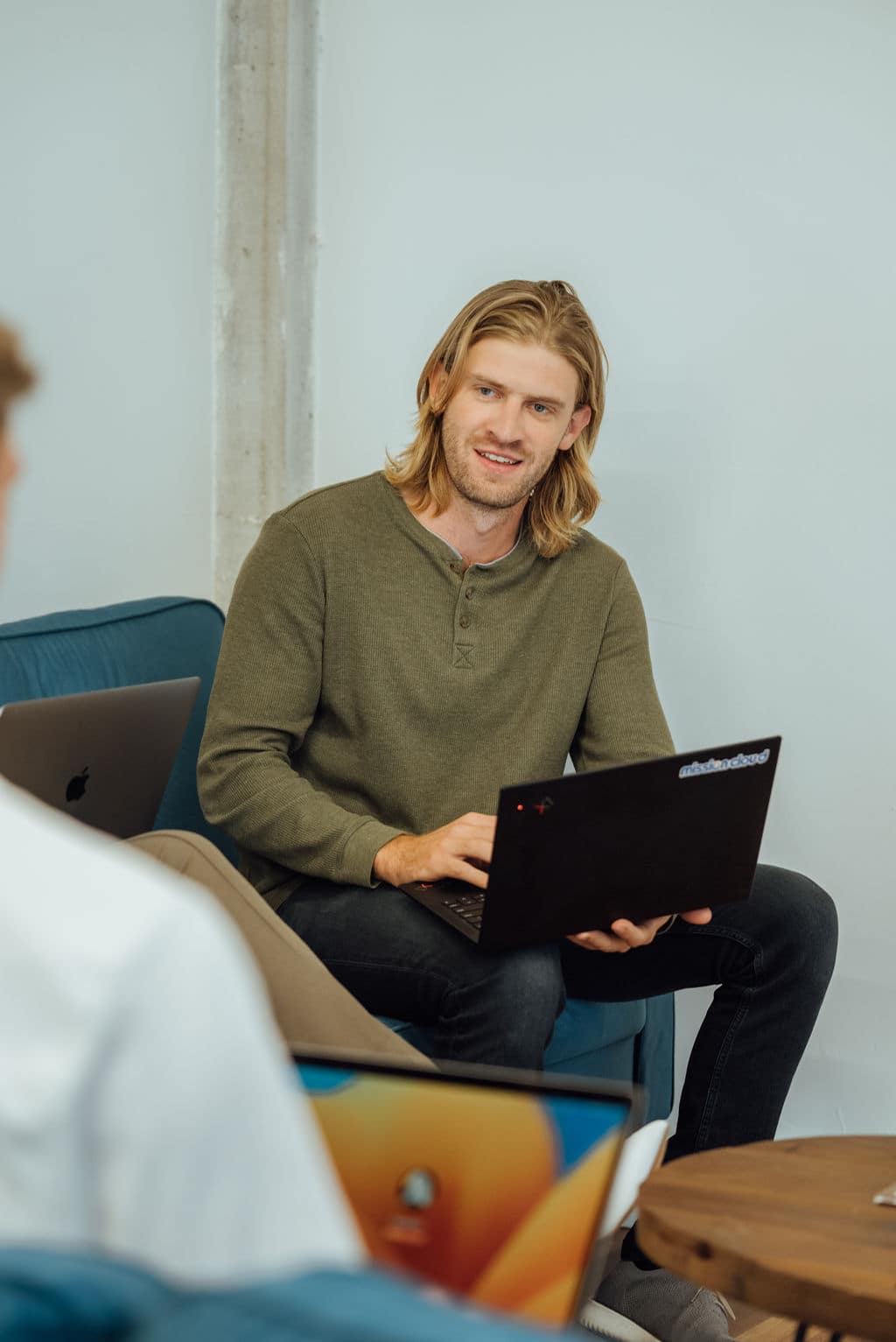
Make Critical Decisions with the Help of Custom ML Models
The practical application of data science can bring value to any business. Working with Mission gives you the expert machine learning consulting you need to model the meaning of your data, understand what it’s telling you, and make decisions in a more informed way. Help your organization improve processes, attract new customers, and anticipate changes in your market.
What AI and Machine Learning Mean For Your Business
Drive Innovation for New Products and Solutions
Uncover issues and deficiencies that might otherwise go unnoticed. Fuel your product development cycle with a better understanding of purchase decisions, consumer feedback and activity, and company processes.
Uncover Business Insights and Trends
With data science and predictive ML models, companies discover previously unknown insights, patterns, and correlations inside their data to improve their business models, operations, and resource management, growing their profitability.
Smarter and Better Responses to Change
By developing models to forecast your market and business conditions, you can predict, anticipate, plan, and respond to changing situations in real time.
Harness the Power of AI/ML Services for Endless Possibilities
Our data science and AI/ML expertise include supervised, unsupervised, and deep learning, and generative AI. We help you find and refine algorithms and models, and we’ll assist you in creating and implementing customized solutions tailored to your specifications and business objectives.
-
ML Models
Develop ML models that feature classification, regression, prediction, clustering, association rules and anomaly detection using supervised, unsupervised, reinforcement, self-learning and mixed-learning algorithms. Learn more about our MLOps Services.
-
Recommendation Engines
Set up ML-driven recommender systems that evaluate historical interactions and characteristics of objects and users to generate reliable, tailored and effective recommendations.
-
Augmented Analytics
Leverage AI and ML to automate and improve analytics at every stage of the data life cycle. Democratize your data and prompt data literacy by enabling more people in your organization to extract meaningful insights from various data sources.
-
Cognitive Solutions
Apply human-like intelligence to tasks with cognitive analytics, and tap into unstructured data sources. Improve innovation and find solutions using cognitive technologies such as computer vision, natural language processing (NLP), speech recognition, text analytics and automation.
-
Deep Learning
Take advantage of deep learning models, which improve over time by recognizing sophisticated patterns in images, text, audio and other data for more precise insights and better predictions. Deep learning on the cloud allows you to design, develop and train deep learning applications faster.
-
ModelOps
Obtain the best possible outcomes for all models in production with ModelOps. ModelOps solutions automate risk management and regulatory compliance processes so that models can be checked and assessed for technical conformance, commercial value, and business and operational risk.
What are the real differences between AI, ML, and deep learning?
You can think of AI as being the broadest topic, which contains the others, because it is generally about making computers perform tasks that require human-like thinking. AI can be a lot of things by that definition. Machine learning is a subset of AI, in which you train models using data to optimize for a specific outcome. This is why ML models often have a predictive element to their solution. Deep learning is itself a subset of ML and uses neural networks with many layers to analyze various factors of data with the aim of improving performance on a given task.
How much will it cost me to train and run ML models on AWS?
It depends. There are a lot of factors, including the scale of your solution, the instance type on which it will best perform, how long you plan to run the solution, and what the supporting data architecture will be. In general, the most reliable way to estimate a cost is to work with an AWS-certified Solutions Architect to work on scoping out the size of what you want accomplished and the most efficient way to engineer it. Since we know this is often on customers’ minds, we offer a free hour with our Data, Analytics, and Machine Learning team to do just that, walking through each part of your solution and even working backward, if necessary, from your desired business outcomes.
How do I know I’ve prepared my data correctly for ML training on AWS?
In terms of collection, you want to find data that’s representative of the real-world scenarios where your ML model will be applied. If your data isn’t really relevant to your domain or representative of its characteristics, you may optimize a model that cannot handle the real-world task. You’ll also need to prepare the data, using services like AWS Data Wrangler, or building a full ETL pipeline through services like AWS Glue. Are you doing supervised or unsupervised training? Supervised training will require labeled data, which can represent its own challenge—consider AWS SageMaker Ground Truth for this. And when you get to engineering your models, consider how you will select features, validate results, experiment, iterate, and reproduce as you train a model. ML engineering is an iterative process, often requiring you to test and adjust frequently until you arrive at a solution.
How can I know whether or not my problem is a good fit for ML?
First, do you have sufficient quality data to train an algorithm? One of the hardest problems can simply be having the scale of data that makes for accurate training. Also, if your problem will shift according to environmental conditions, like macroeconomics, for example, this can be a better fit for ML’s adaptability than other ways of solving. In general, prediction-oriented problems with a large historical data set are a good fit. And problems which involve hard-to-identify patterns are where ML shines, by detecting a pattern that would otherwise be difficult to pin down with other analytic methods.
It seems like there's always a lot of hype around AI and ML—is there real business value here?
It depends on your business but there are many well-established use cases for machine learning. Identifying consumer sentiment, trend analysis and forecasting, recommendation engines, fraud detection, healthcare diagnostics, manufacturing monitoring—all of these have seen considerable advances from machine learning solutions. A healthy dose of skepticism is warranted—the media often has trouble distinguishing between real use cases and companies looking to brand themselves—but if your problem has a strong fit for ML techniques there can be large upside to a well-architected solution.
Which technology should I use: generative AI, ML, or traditional AI?
This depends on your problem, goals, and the availability of data for your solution. Generative AI is strong for creating novel content, like prototyping, or the synthesis of several data sources, or even a simulation of some real world phenomena. Machine learning is more narrowly about solving problems by absorbing and iterating over a large amount of data for solutions that need to predict outcomes, identify patterns, or adapt to a shifting environment. Traditional AI is rule-based and best when the problem has clearly definable logic but presents too many variables for other programmatic solutions.
How can machine learning benefit my business?
Machine learning consulting can empower your business by turning complex data into actionable insights, helping you streamline operations, personalize customer experiences, and discover new growth opportunities. Ultimately, machine learning helps your organization stay agile, reduce operational costs, and consistently deliver value to customers.
What Our Clients Are Saying
“It became evident from day one that Mission wasn’t just going to throw something over the fence for us to use; the team was going to ensure that we understood the rationale behind the processes and technologies put into action.”
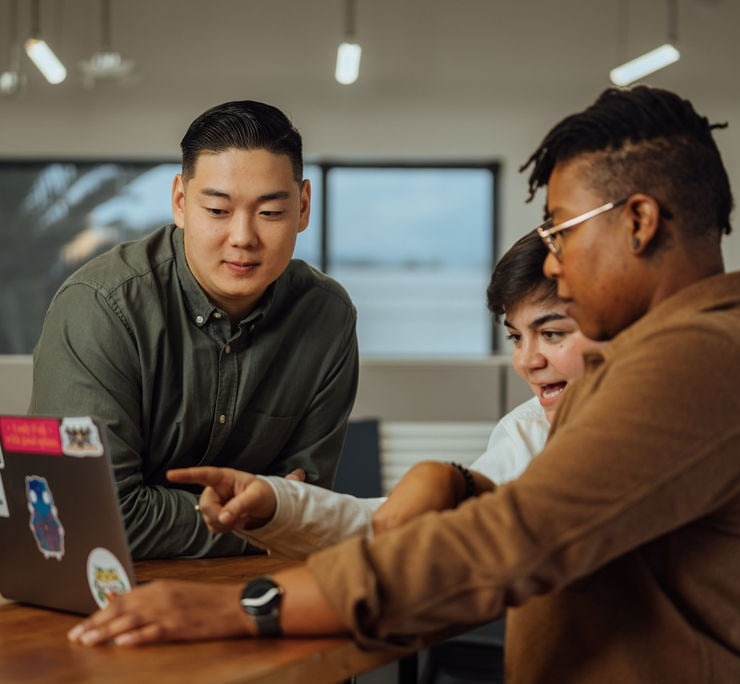
Get in touch
Learn How We Can Help With Data Science and AI/ML on AWS
Leveraging AI/ML through AWS has never been easier. Get in touch to learn how we provide you with the groundwork you need to realize all the advantages that data science and AI/ML offer.